16 Apr 2019
Deep Inverse Sensor Modelling in Radar
Image
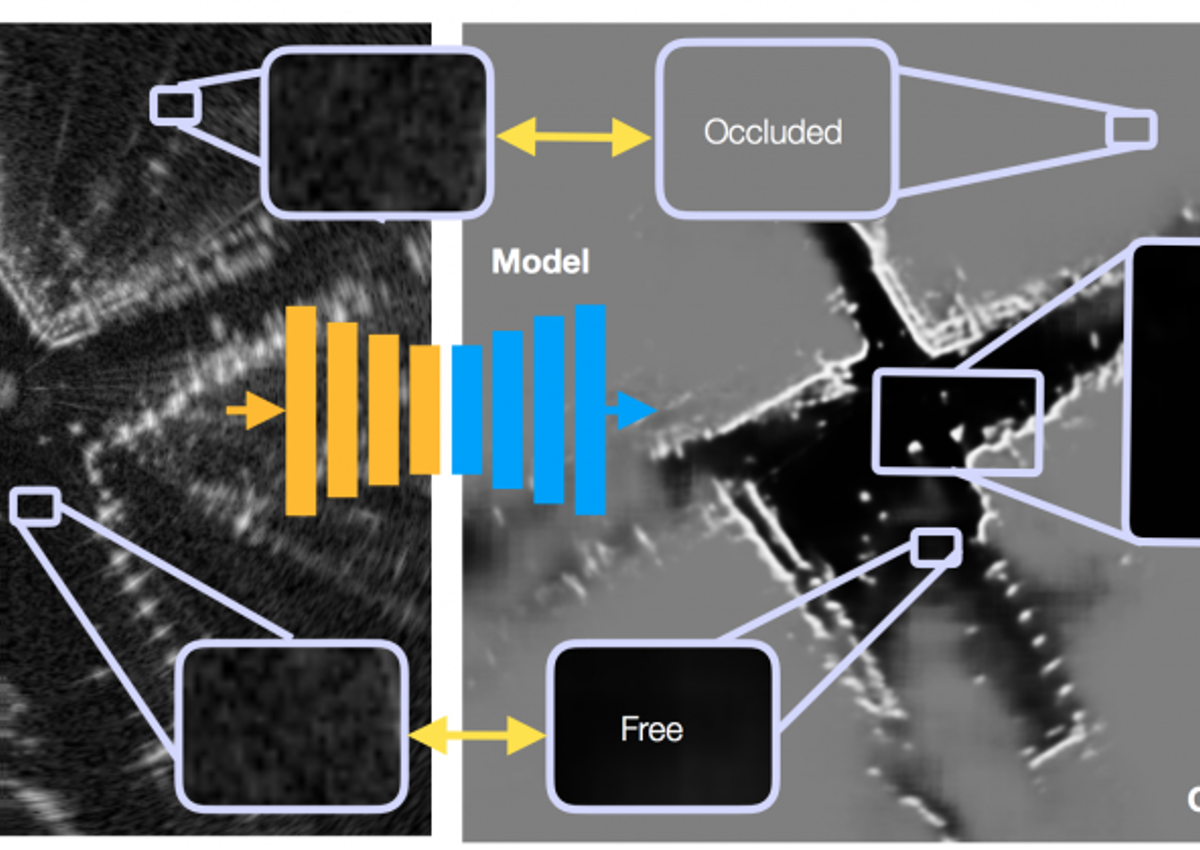
In the last decade, systems utilising camera and lasers have been remarkably successful increasing our expectations for what robotics might achieve in the decade to come. Our robots now need to see further, not only operating in environments where humans can operate, but also in environments where humans cannot! To this end radar is a promising alternative to laser and vision being able to detect objects at far range under a variety of challenging conditions (such as dust and blizzards). However the complex interaction between aleatoric noise artefacts and occlusion makes interpreting raw radar data challenging. Our primary observation is that as humans, our uncertainty in the distinction between occupied and free space varies throughout the scene as a function of scene context: Low power returns in front of walls are more likely to correspond to free space than low power returns behind. Inspired by this we utilise a heteroscedastic noise model in combination with a deep neural network to learn to predict space as free or occupied whilst explicitly allowing the uncertainty in this prediction to vary as function of scene context. Our model is self-supervised utilising the detections made in laser mounted to the same vehicle, allowing us to train our model by simply traversing an environment. Through our approach we are able to convert noisy radar scans to a grid of occupancy grid probabilities, outperforming classical filtering methods at detecting true objects. Simultaneously, thorough our heteroscedastic noise model we are able to simultaneously identifying space that is likely to be occluded. For more information please see our most recent paper!