Science
Science
Research at the MRG is concerned with building and inventing the next generation of competencies for autonomous vehicles:
- Using ultra-low-cost mapping for vast scale localisation
- Developing Weather immune perception and localisation with advanced sensor technologies and techniques which "edit out" the weather from sensor streams
- Building perception systems which are suitable for autonomous vehicle systems satisfying emerging safety standards.
- Moving mobile robotics compute away from the edge (vehicles) and into the cloud and IOT technology,
- Building hybrid systems which leverage the best of classical and deep learning techniques
On the Road: Route Proposal from Radar Self-Supervised by Fuzzy LiDAR Traversability

On the Road: Route Proposal from Radar Self-Supervised by Fuzzy LiDAR Traversability
This paper uses a fuzzy logic ruleset to automatically label the traversability of the world as sensed by a LiDAR in order to learn a Deep Neural Network (DNN) model of drivable routes from radar alone.
kRadar++: Coarse-to-fine FMCW Scanning Radar Localisation

kRadar++: Coarse-to-fine FMCW Scanning Radar Localisation
This paper presents a hierarchical approach to place recognition and pose refinement for Frequency-Modulated Continuous-Wave (FMCW) scanning radar localisation.
Self-Supervised Localisation between RangeSensors and Overhead Imagery

Self-Supervised Localisation between RangeSensors and Overhead Imagery
This paper shows how to use satellite imagery as a ubiquitus and cheap tool for vehicle localisation with range sensors through a self-supervised pipeline, i.e. without a metrically accurate ground-truth signal.
Keep off the Grass: Permissible Driving Routes from Radar with Weak Audio Supervision
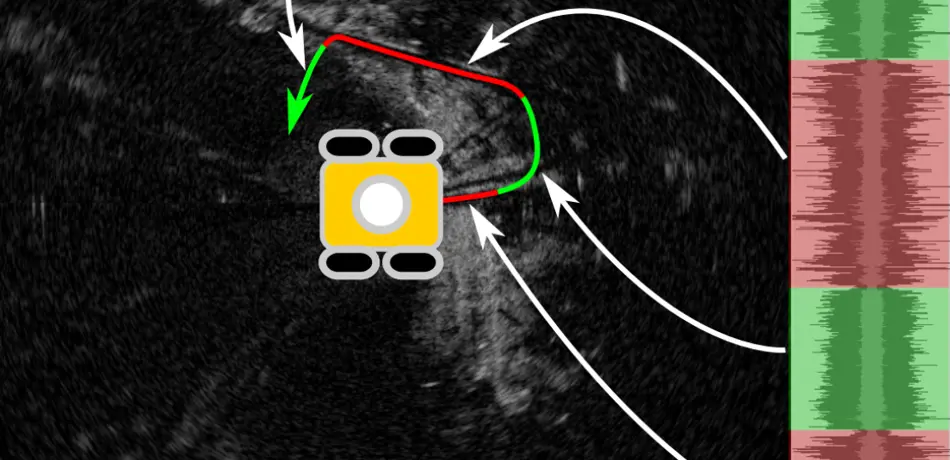
Keep off the Grass: Permissible Driving Routes from Radar with Weak Audio Supervision
Learning to segment a radar scan based on driveability in a fully supervised manner is not feasible as labelling each radar scan on a bin-by-bin basis is both difficult and time-consuming to do by hand. We therefore weakly supervise the training of the radar-based classifier through an audio-based classifier that is able to predict the terrain type underneath the robot.
Sense-Assess-eXplain (SAX): Building Trust in Autonomous Vehicles in Challenging Real-World Driving Scenarios

Sense-Assess-eXplain (SAX): Building Trust in Autonomous Vehicles in Challenging Real-World Driving Scenarios
In this paper, we discuss the ongoing work under the Sense-Assess-eXplain (SAX) founded project. We analyse our research methodology as well as describe ongoing work in the collection of an unusual, rare, and highlyvaluable dataset.
RSS-Net: Weakly-Supervised Multi-Class Semantic Segmentation with FMCW Radar
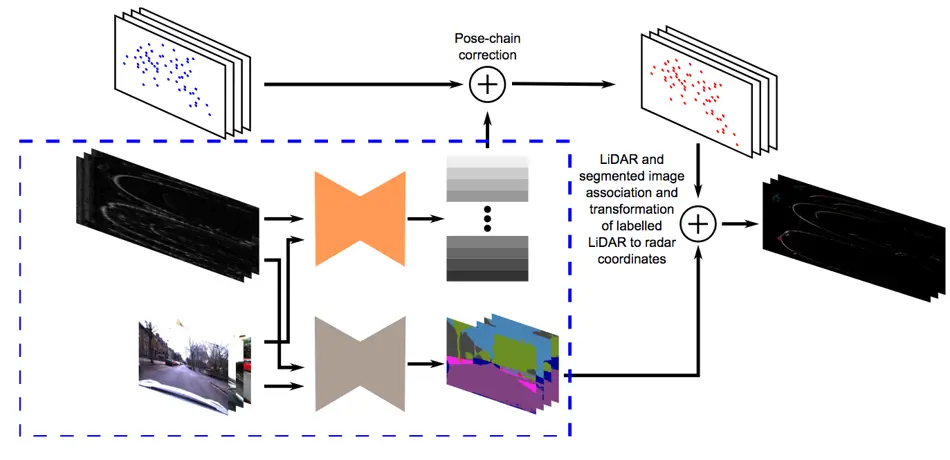
RSS-Net: Weakly-Supervised Multi-Class Semantic Segmentation with FMCW Radar
Manual labelling for segmentation tasks is very time-consuming; moreover, radar has an intrinsically complex behaviour, leading to observations which make annotation difficult even for expert users. Here we propose a method to label radar data exploiting well-known image and lidar pipelines.
LiDAR Lateral Localisation Despite Challenging Occlusion from Traffic
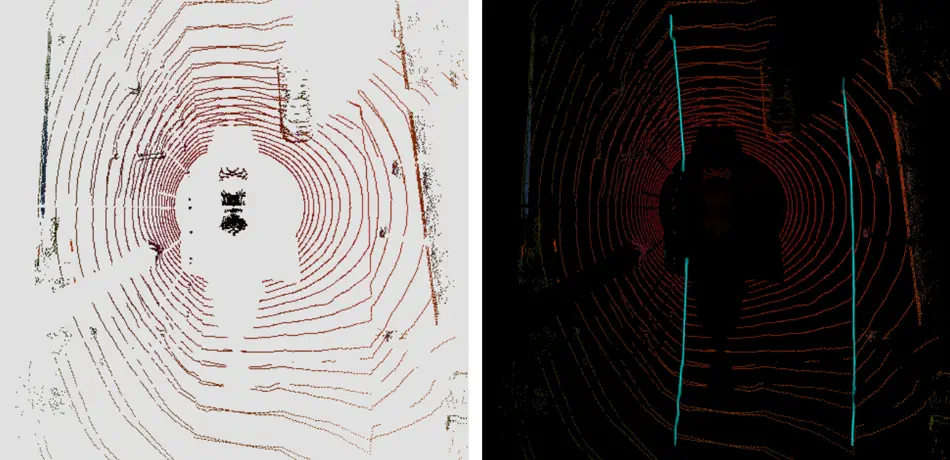
LiDAR Lateral Localisation Despite Challenging Occlusion from Traffic
We show in this paper how we can improve the robustness of LiDAR lateral localisation systems by including detections of road boundaries which are invisible to the sensor (due to occlusion, e.g. traffic) but can be located by our Occluded Road Boundary Inference Deep Neural Network.
Look Around You: Sequence-based Radar Place Recognition with Learned Rotational Invariance
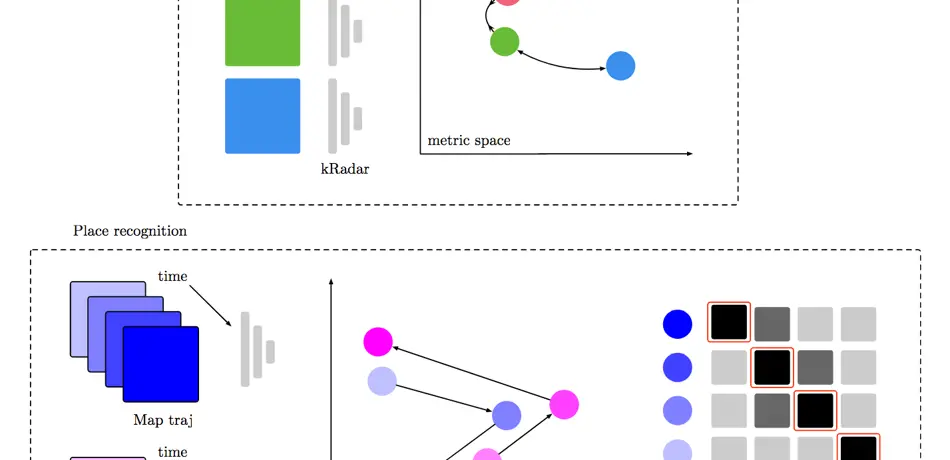
Look Around You: Sequence-based Radar Place Recognition with Learned Rotational Invariance
This paper presents the integration of a rotationally-invariant metric embedding for radar scans into sequence-based trajectory matching systems and how this procedure improves the place recognition task in a teach-and-repeat scenario.
Real-time Kinematic Ground Truth for the Oxford RobotCar Dataset
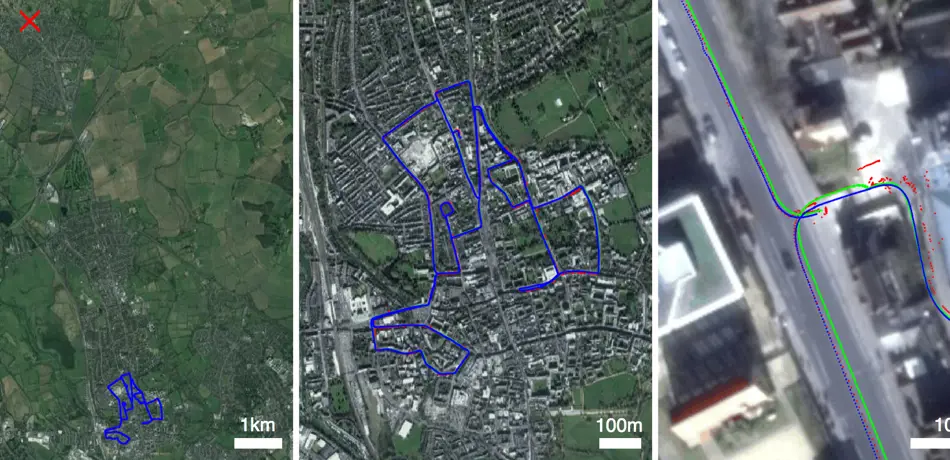
Real-time Kinematic Ground Truth for the Oxford RobotCar Dataset
In this paper, we describe the release of globally-consistent centimetre-accurate reference data towards a challenging long-term localisation and mapping benchmark based on the large-scaleOxford RobotCar Dataset through the usage of post-processed raw GPS, IMU, and static GNSS base station recordings.
RSL-Net: Localising in Satellite Images From a Radar on the Ground
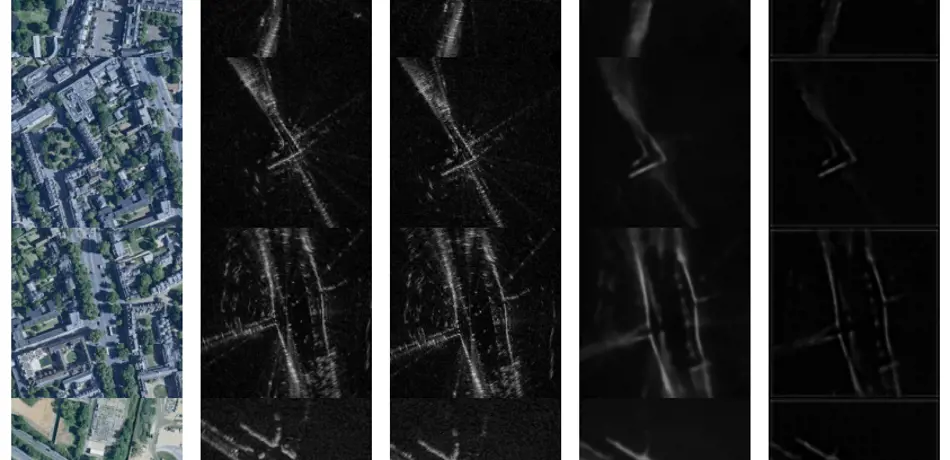
RSL-Net: Localising in Satellite Images From a Radar on the Ground
In this paper we propose a learnt methodology for exploiting overhead imagery, e.g. satellite images from well-known online services, as cheap localisation data for a vehicle equipped with a radar sensor.
Distant Vehicle Detection Using Radar and Vision

Distant Vehicle Detection Using Radar and Vision
To mitigate performance drops of neural networks in a detection task falls when detecting small (distant) objects, we propose to incorporate radar data to boost performance in these difficult situations.
Kidnapped Radar: Topological Radar Localisation using Rotationally-Invariant Metric Learning
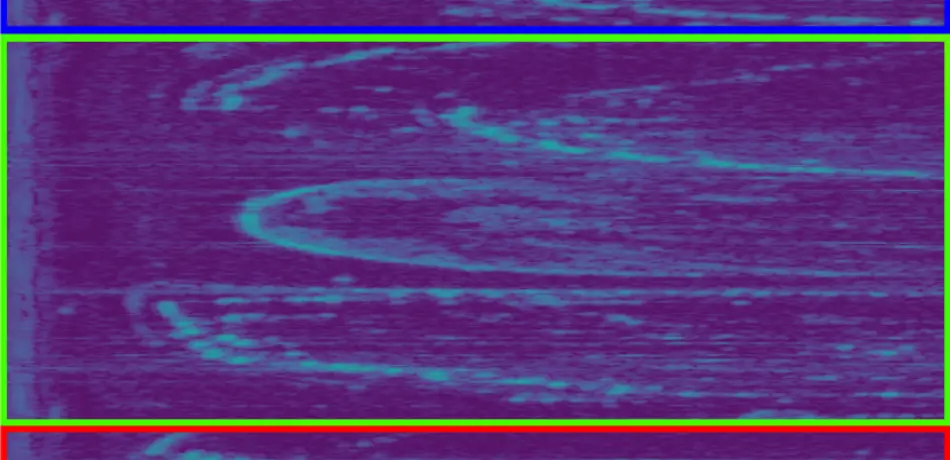
Kidnapped Radar: Topological Radar Localisation using Rotationally-Invariant Metric Learning
This paper presents a system for robust, large-scale topological localisation using Frequency-Modulated ContinuousWave (FMCW) scanning radar, throught the learning of a rotationally-invariant, multidimensional embedding space.
I Can See Clearly Now : Image Restoration via De-Raining
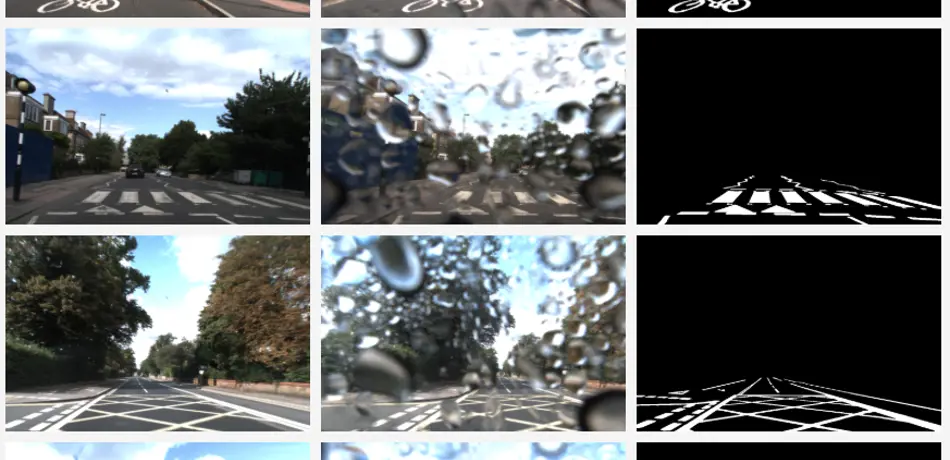
I Can See Clearly Now : Image Restoration via De-Raining
By supervising a denoising generator using a custom dataset composed of pairs of images where one lens is affected by real water droplets while keeping another lens clear, we demonstrate we can improve segmentation tasks on images affected by adherent rain drops and streaks.
The Hulk: Design and Development of a Weather-proof Vehicle for Long-term Autonomy in Outdoor Environments

The Hulk: Design and Development of a Weather-proof Vehicle for Long-term Autonomy in Outdoor Environments
In this paper we describe the design choice that led us to the development of the Hulk, our new weather-proof platform for investigating long-term autonomy in outdoor environments.
What Could Go Wrong? Introspective Radar Odometry in Challenging Environments

What Could Go Wrong? Introspective Radar Odometry in Challenging Environments
This paper is about detecting failures under uncertainty and improving the reliability of radar-only motion estimation by weakly supervision of a classifier that exploits the principal eigenvector associated with our radar scan matching algorithm at run-time.
The Right (Angled) Perspective: Improving the Understanding of Road Scenes Using Boosted Inverse Perspective Mapping

The Right (Angled) Perspective: Improving the Understanding of Road Scenes Using Boosted Inverse Perspective Mapping
Many tasks performed by autonomous vehicles such as road marking detection, object tracking, and path planning are simpler in bird’s-eye view. In this paper, we present an adversarial learning approach for generating a significantly improved IPM from a single camera image in real time.
Fast Radar Motion Estimation with a Learnt Focus of Attention using Weak Supervision
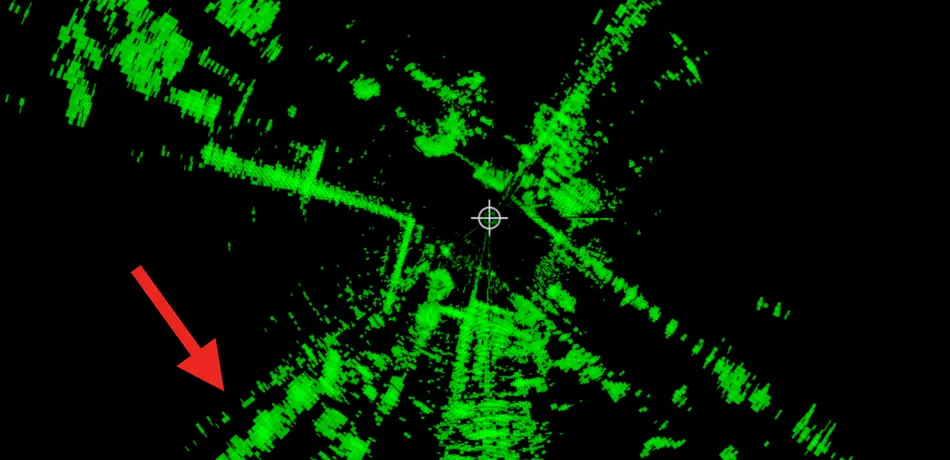
Fast Radar Motion Estimation with a Learnt Focus of Attention using Weak Supervision
We use weak supervision to train a focus-of-attention policy which actively down-samples the measurementstream before data association steps are undertaken in our radar-only motion-estimation pipeline for bolster its speed without effecting the accuracy.
Radar-only ego-motion estimation in difficult settings via graph matching

Radar-only ego-motion estimation in difficult settings via graph matching
In this work, we propose a radar-only odometry pipeline that is highly robust to radar artifacts, demonstrating adaptability across diverse settings, from urban UK to off-road Iceland.
Inferring Road Boundaries Through and Despite Traffic

Inferring Road Boundaries Through and Despite Traffic
This paper is about the detection and inference of road boundaries from mono-images irrespective of whether or not the boundary is actually visible, by approaching it as a coupled, two-class detection problem, i.e. solving for occluded and non-occluded curve partitions with a continuity constraint.
Precise Ego-Motion Estimation with Millimeter-Wave Radar under Diverse and Challenging Conditions

Precise Ego-Motion Estimation with Millimeter-Wave Radar under Diverse and Challenging Conditions
In this paper, we present a reliable and accurate radar-only motion-estimation algorithm for mobile autonomous systems, showing good performances under variable weather and lighting conditions and without an external infrastructure.
Geometric Multi-Model Extraction For Robotics

Geometric Multi-Model Extraction For Robotics
In this paper, we propose a novel method for fitting multiple geometric models to multi-structural data via convex relaxation through the minimisation of the energy of theoverall assignment. The inherently parallel nature of our approach allows for the elegant treatment of a scaling factor that occurs as the number of features in the data increases.
Meshed Up: Learnt Error Correction in 3D Reconstructions
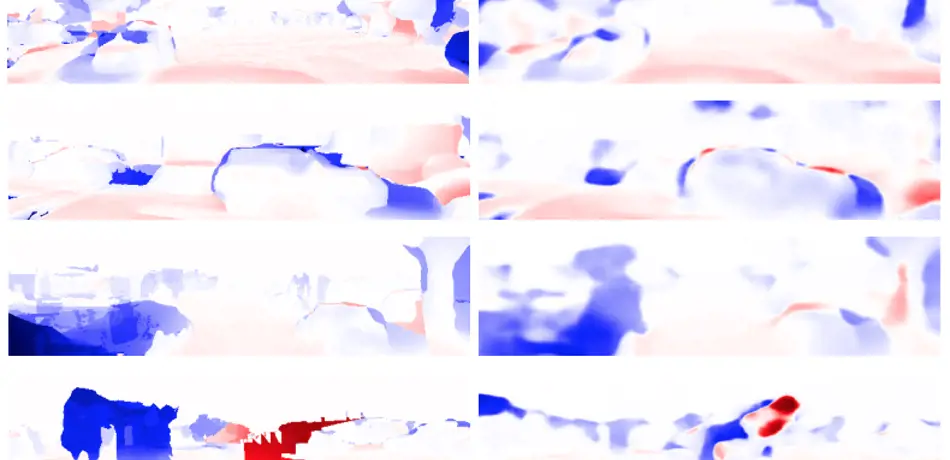
Meshed Up: Learnt Error Correction in 3D Reconstructions
This paper proposes a machine learning technique to identify errors in three dimensional (3D) meshes enabling us to improve the reconstruction accuracy. We train a suitably deep network architecture supervising the task of correcting lower-quality, stereo-image reconstructions using high-quality laser reconstructions.
Mark Yourself: Road Marking Segmentation via Weakly-Supervised Annotations from Multimodal Data
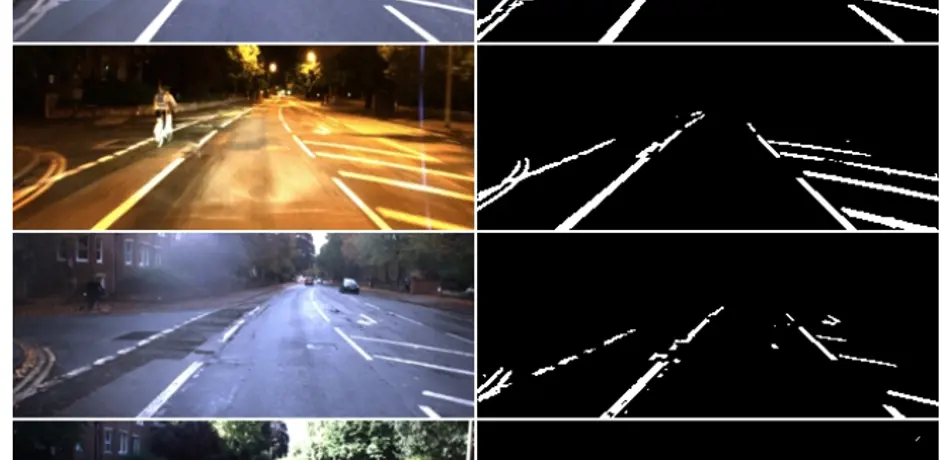
Mark Yourself: Road Marking Segmentation via Weakly-Supervised Annotations from Multimodal Data
This paper presents a learnt system for real-time road marking detection from images obtained from a monocular camera trained through weakly-supervision by exploiting additional sensor modalities to generate large quantities of annotated images.